Ones and Zeros
The rise and rise and rise of data.
How Everything Became Data
Starting with the birth of statistics in the 19th century and concluding with algorithms and AI systems, a new book examines how humans became studied as a set of ones and zeroes.
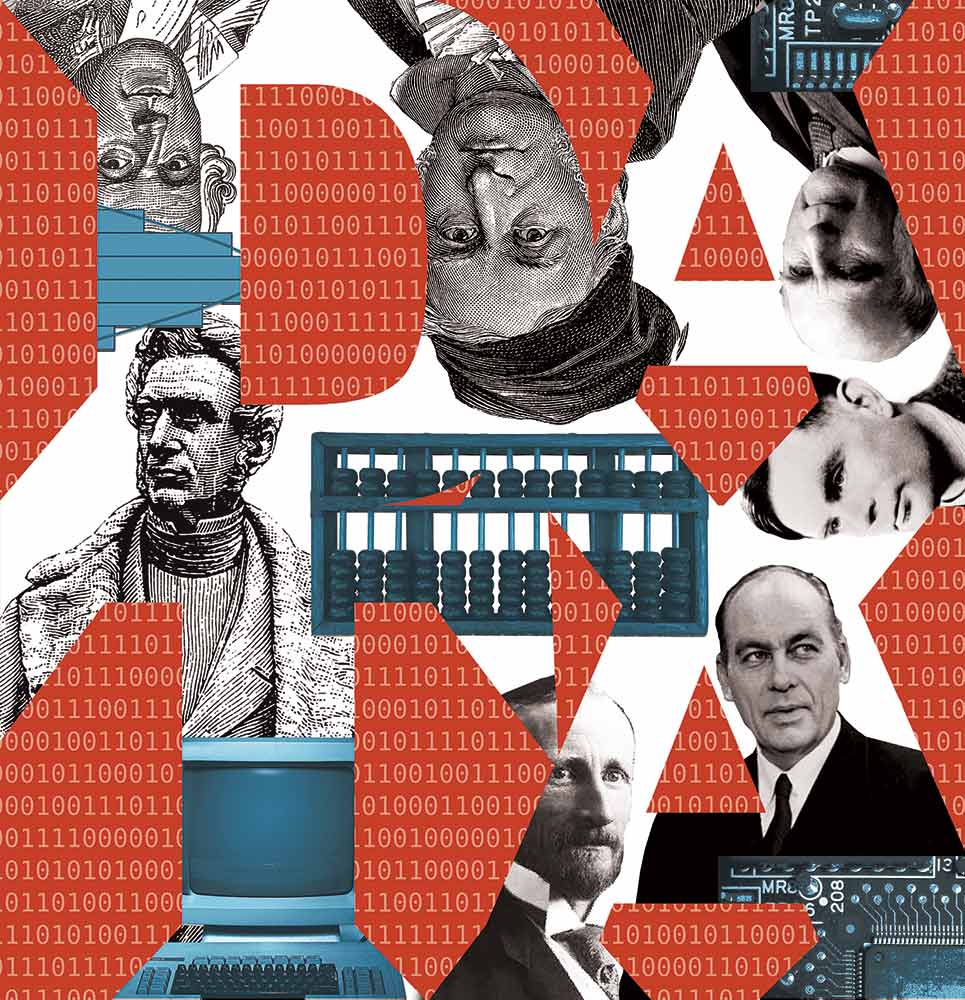
Some words are more important than others, Raymond Williams once observed; they carry more of our common life, and therefore their meanings are denser and more complex. But a paradox ensues: The words we find most indispensable are also the ones we find hardest to define.
“Data” today is one of those words. You could go to the dictionary, but that wouldn’t get you very far. To say that data is information, typically in digital form, says nothing about the strength or the strangeness of the psychic investments summoned by those four letters. We talk about data the way the 19th-century bourgeois often talked about culture: There is an airplane hangar’s worth of symbolic meanings evoked every time we utter the term.
Books in review
How Data Happened: A History From the Age of Reason to the Age of Algorithms
Buy this bookWhen we talk about data, we tend to be talking about optimization as well. Optimization is what data promises. By representing reality with numbers and then using statistical techniques to analyze them, we can make reality more efficient and thus profitable. Relationships are revealed that have an explanatory power: for example, which people are most likely to click on a particular ad, or which financial asset is most likely to increase in value. This capability is sometimes discussed as “artificial intelligence,” but more prosaically it’s inference, albeit on an industrial scale.
Making inferences from data is an important feature of capitalism. It helps predict the future behavior of consumers, borrowers, and workers in potentially lucrative ways. For this reason, data is now being generated about every aspect of human life in every part of the world. This wasn’t always the case, but it’s hard to say exactly when it started. You might think the answer would be straightforward, given that computers clearly date from a certain decade (the 1940s). But their invention and use wouldn’t have been possible without a long prehistory of analog computation.
Chris Wiggins and Matthew L. Jones’s new book, How Data Happened: A History From the Age of Reason to the Age of Algorithms, chronicles how human beings became data. Starting with the birth of modern statistics in the 19th century and concluding with the statistics-based AI systems of the present day, the authors—an assistant professor of applied mathematics at Columbia University and a professor of history at Princeton, respectively—aim “to provide a framework for understanding the persistent role of data in rearranging power.” Data-driven processes, they argue, are responsible for innumerable injustices, from reinforcing racist policing to facilitating the routine humiliation of welfare beneficiaries. To undo the damage done by these processes requires “concentrated political action,” and such action in turn requires “a clear understanding of how contingent—how not set in stone—our current state of affairs is.” Much like those historians who try to figure out how and when capitalism started in the hopes of helping bring it to an end, Wiggins and Jones hope to historicize our datafied social order in order to hasten its replacement with a more humane alternative, one in which data is used in defense of people’s dignity.
How Data Happened begins in the early 19th century with the Belgian astronomer Adolphe Quetelet. One could begin earlier, of course: For millennia, human beings have collected information about the world and encoded it into art and artifacts. But what interests Wiggins and Jones is a specific intellectual tradition that underlies today’s algorithmic systems—a tradition that Quetelet helped found. Living at a time of rapid progress in celestial mechanics, the Belgian had begun to use new statistical techniques developed by the French mathematician and astronomer Pierre-Simon Laplace and the German mathematician and physicist Carl Friedrich Gauss to study the position of stars. Soon Quetelet proposed enlisting these techniques in the study of society and social behavior as well. Through an analysis of numbers about such things as height, death, and crime, he argued, the hidden order of human life would be revealed.
Fortunately for Quetelet, the burgeoning imperial states of Europe had begun collecting information about their population and resources in order to expand tax collection. Wars were expensive, and data could help find ways to pay for it. Quetelet wrangled some of this information from officials he knew in Belgium, the Netherlands, and elsewhere in Europe, as well as from fellow statisticians who were compiling their own collections. Creating averages out of the information, he then developed general theories about humanity, treating these averages “as if they were real quantities out there,” Wiggins and Jones write. Quetelet’s averages were not merely descriptive but prescriptive; the average man also became the ideal one. “If the central region of the bell curve was normal,” the authors continue, “its peripheries were evidently the realm of the pathological.”
So long as we remember that such measures are abstractions, they can be useful. But as Wiggins and Jones point out, the Queteletian fantasy that the map is the territory has proved remarkably persistent throughout the history of data. For example, crime rates are a set of numbers about the recorded incidence of activities considered worthy of policing. Yet a number of assumptions have already worked their way into the data at the moment of its making: what gets recorded, who does the policing and who gets policed, and, perhaps most important, what is considered a crime. Even in the aggregate, these numbers do not represent reality in its fullness, but they nonetheless take on a life of their own. By shaping politicians’ perceptions of a city or neighborhood or group of people, and by training the algorithmic policing software that predicts who will commit a crime and where crimes will be committed, they create new realities on the ground: Particular neighborhoods are saturated with cops, and particular groups are turned into targets of policing. More and more criminals are the result.
Although these innovations would occur only in the 20th and 21st centuries, the statisticians in Quetelet’s time were already highly susceptible to the seductions of abstraction. Indeed, the Belgian’s tendency to confuse the abstract with the concrete became a major feature of the field he helped create. Another was racism. The Victorian polymath Francis Galton, who drew on Quetelet’s work, was an emblematic case. Galton was a eugenicist—in fact, he coined the term—and believed that data analysis would provide a scientific basis for racial hierarchy. Along the way, he developed methods like correlation and regression that remain foundational to the practice of statistics.
From the start, therefore, statisticians helped make policy, and those policies created a set of self-fulfilling prophecies. Wiggins and Jones recount the case of the German émigré statistician Frederick Hoffman, who was hired by the Prudential insurance company in the late 19th century. Hoffman concluded that Black people’s higher mortality rates indicated their essential biological inferiority; therefore, they deserved to be charged higher life insurance premiums or denied coverage altogether. As Black breadwinners died, their families were left without a safety net, contributing to the kinds of hardship that caused Black people to die sooner than white people. Thus Hoffman helped produce the social facts that lay beneath the data he analyzed. Ever since Quetelet brought statistical thinking from the stars down to earth, Wiggins and Jones observe, the discipline has not “simply represent[ed] the world…. It change[d] the world.” And mostly, it seems, for the worse.
In the early 20th century, the power and prestige of statistics grew. Government records like birth certificates and censuses became more detailed and systematic, turning “people into data in a hitherto unprecedented way,” the authors write. Intelligence testing took off during World War I, as a way to evaluate recruits. Before the 1936 presidential election, George Gallup used the statistical technique of survey sampling to predict Franklin Roosevelt’s victory, inaugurating the era of modern opinion polling. Statisticians also played an indispensable role in the New Deal, as their analyses of industrial and agricultural data helped guide the administration’s economic planning. But the real turning point came in the 1940s, with the invention of the digital electronic computer. Although statistical thinking had been influential before this point, it would soon become inescapable.
Modern computing has many birthplaces, but one of them is Bletchley Park, the English country estate where, during World War II, the mathematician Alan Turing and his colleagues deciphered top-secret Nazi communications encrypted with the Enigma machine. In the process, they would create machines of their own: first specialized electromechanical devices called “bombes,” then a programmable computer named Colossus. These inventions mechanized the labor of statistical analysis by enabling the code breakers to make educated guesses—at high volume and speed—about which settings had been used on the Enigma machine to encode a given message and then to refine those guesses further as more information became available. “Bletchley mattered because it made data analysis industrial,” Wiggins and Jones write. The quantification of the world could advance only so far by hand. Now there was a vast infrastructure—a forest of twinkling vacuum tubes, and later microchips—to help move it to the next stage.
The significance of these efforts continued to increase during the Cold War. Like the empires of Quetelet’s day, the American empire that emerged in the postwar era saw data as integral to both its dominance in the West and its struggle for power over the rest of the world. The National Security Agency, founded in 1952, became the centerpiece of these efforts. “By 1955, more than two thousand listening positions produced thirty-seven tons of intercepted communication that needed processing per month, along with 30 million words of teletype communications,” the authors write. The technology for storing and processing such an abundance of data didn’t exist, so the NSA funded it into existence. The agency paid tech companies to create storage media in order to keep pace with its growing cache of intercepts and pioneered new kinds of computational statistics to interpret it all.
The animating problem for the NSA was this tsunami of data and the need to make it meaningful. And this problem, while initially posed by the public sector, ended up generating some lucrative lines of business for the private sector. Corporate America began to embrace computing in the 1960s. Within a decade, there were databases filled with the personal information of millions of Americans. Credit card purchases, air travel, car rentals—each data point was dutifully recorded. Computational statistics began to inform business decisions such as who to give a loan and who to target in a marketing campaign.
Popular
“swipe left below to view more authors”Swipe →Mass digitization provoked resistance as well. Wiggins and Jones describe the political debate over personal information that erupted in the 1970s, propelled in part by Watergate. In 1974, Senator Sam Ervin, who had served as chair of the Senate Watergate Committee, introduced a bill that “aimed to establish control of personal data as a right of every American citizen,” Wiggins and Jones write. Crucially, the bill’s provisions covered both government and corporate databases, and the discussions in Congress weren’t just about privacy but also justice—that is, about “the potential harms of automatic decision making and the likely disproportionate impact upon less empowered groups.”
Such harms may sound all too current, but they have been with us for some time. Take credit scores: Digitizing customer data made it possible to do computerized assessments of creditworthiness via statistical modeling. While this may seem more objective than leaving things to a loan officer at the local bank, it created a powerful new vector for racial oppression in the post-civil-rights era. Software made racism mathematical, obscuring its operation while magnifying its effect.
Ervin’s 1974 bill garnered bipartisan support, but business hated it. Wiggins and Jones quote a scaremongering statement from one credit card company: “If the free flow of information is impeded by law, the resulting inefficiencies will necessarily be translated into higher costs to industry and consumer.” Under pressure, Congress shrank the scope of the proposed bill. The version that passed, the Privacy Act of 1974, applied only to the federal government. Wiggins and Jones describe subsequent laws that placed some limited controls on corporate data practices, most notably with the Health Insurance Portability and Accountability Act (or HIPAA) in 1996, which restricts how healthcare companies can use patient information. Yet the private sector was mostly left alone. The relative absence of regulation enabled the development of increasingly data-intensive business models in the last quarter of the 20th century, culminating with the rise of the platform economy in the 2000s and 2010s.
This capitulation on corporate data gathering represented a major missed opportunity, in Wiggins and Jones’s view. “Following this failure to protect nongovernmental data, the free use and abuse of personal data came to seem a natural state of affairs—not something contingent, not something subject to change, not something subject to our political process and choices,” they write. Datafication is as much a political project as a technical one, the authors remind us. Its current dystopian form is in large part the legacy of concrete political failures.
How Data Happened does a lot of things well. Difficult concepts are deftly untangled. The racist lineage of statistics is dealt with unsparingly. The twin temptations of tech triumphalism and catastrophism are successfully resisted; the tone is sober throughout. The book provides a useful new vantage point on the history of computing, one that puts data at the center.
Yet the book doesn’t quite hold together from start to finish. It is based on an undergraduate class that the authors taught at Columbia from 2017 until recently; most chapters correspond to particular lectures. This gives the material an episodic quality that is probably well suited to a classroom setting but feels a little disjointed when presented in book form. There are not quite enough through lines, callbacks, and other threads of connective tissue to stitch the text together. The analysis suffers as a result: The history of statistics and the history of computing are linked too loosely in the book for the reader to get a satisfying account of their interaction. For example, it’s significant that racists like Galton contributed so centrally to the development of statistical methods that are now embedded in automated systems that perpetuate racial hierarchies in various ways. There are continuities here to be explored that could have given this book more cohesion.
Beyond the structure of How Data Happened, there is also the matter of structure in it—that is, the role that structural forces play in its narrative. The authors frequently point out that there is nothing inevitable about the course that certain technologies took; rather, those paths were the product of specific choices, ones that could’ve been made differently. This is undeniably true, but it is also only half the truth. Choices are always made within particular sets of constraints and incentives—which to say, they are always constrained and incentivized by particular structures. How was the emergence of statistics as a social science in the 19th century conditioned by the rise of industrial capitalism and the era’s colonial projects? How did US imperialism and mid-century modes of accumulation set the parameters for the growth of data processing in the postwar period? And how do these legacies persist in the present, as mediated through new configurations of empire and capital?
How Data Happened is good at dismantling the technological determinism that the culture sets as our default. But sometimes, in the exhilaration of the jailbreak from determinism, one arrives at a kind of voluntarism. “Militarism and capitalism didn’t simply cause the shift to computer data processing,” the authors write, which of course is true: Causality in such matters is never simple. However, without sufficient attention paid to the structural forces in the causal mix, history can start to look like too frictionless a medium for human action. And this introduces a practical danger, in the same way that flying an airplane without an appreciation for the specific frictions of air as a medium would be dangerous. If the past appears freely chosen—a sequence of what people have willed—then we may misjudge the scale of what’s required to make a better future.
How Data Happened ends with a discussion of the possible routes toward that better future. Wiggins and Jones recommend finding an equilibrium between “corporate power,” “state power,” and “people power” that is capable of making “data compatible with democracy.” A range of strategies are presented, including new regulations, stronger antitrust enforcement, tech worker activism, and the channeling of intercorporate rivalries into the public interest. This is slow, difficult work, they caution, with no quick and simple fixes.
But what precisely needs to be fixed? The authors propose a granular style of politics that preserves the basic contours of our social order while rebalancing the allocation of power somewhat. If the damaging aspects of data are due to a misalignment between new technologies and “our values and norms,” as Wiggins and Jones contend, then such an approach is probably enough. If there are deeper systems at work, however—if computerized inequalities are conjoined to capitalism, imperialism, racism, and their centuries-long entanglements, which together constitute the core logic of our society—then a different kind of politics is needed. This politics may borrow from the strategies the authors present, and it may at times be just as slow. But it will be a politics that aspires to change the context in which choices are made, by building new structures that are proportionate to the structures that have to be undone.
What might such a politics look like in practice? In 1948, while staying in a Reno boardinghouse waiting for his divorce to go through, the historian C.L.R. James passed the time by reading Hegel. “The new thing LEAPS out,” he wrote. “You do not look and see it small and growing larger. It is there, but it exists first in thought.” For James, another world was not only possible but already present in some form. This idea can help us find the prefigurative fragments of a different future all around us. For example, truckers are using mallets to destroy the onboard electronic monitors installed by their bosses. Communities are building democratically governed broadband networks. Gig workers are overcoming app-based alienations to coordinate collective action. If you connect the dots, the provisional shape of an alternative technological paradigm comes into view. There is a pattern in the data, but it exists first in thought. To bring it from thought into material existence involves struggle, as well as an appreciation of the historical conditions within which one is struggling. These conditions may be more or less favorable, but there is some comfort in knowing that resistance always persists—and with it, the everyday solidarities from which a new society can be inferred.